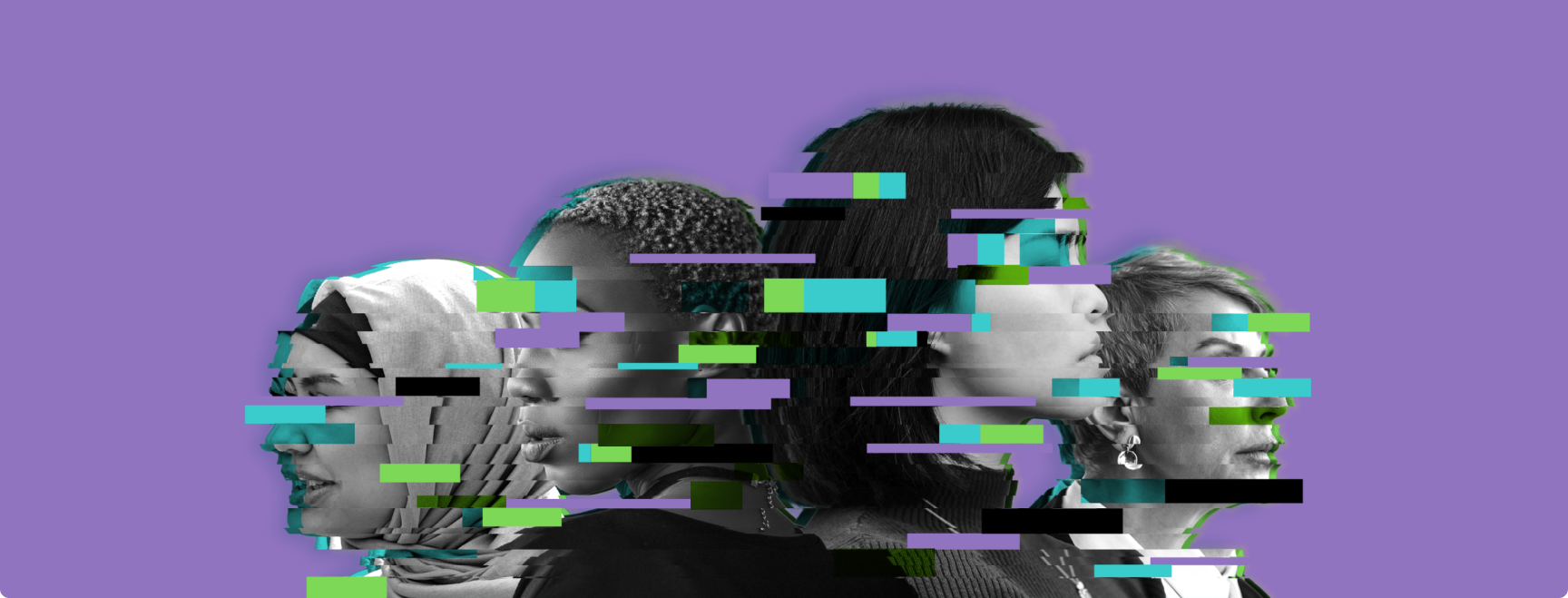
Women in AI: Insights from top leaders
The promise of AI is immense. So is its potential to perpetuate bias. Here's what to look for when writing about AI.
AI is everywhere: news headlines, our phones, doctor’s offices, and banks. Its potential is immense, as are its opportunities for innovation. But AI also raises ethical questions, and presents risks of feedback loops that reinforce existing inaccuracies and biases.
That’s why anyone writing about AI — including our clients who create thought leadership content about it — should do it with eyes open. Content marketing won’t solve the bias-in-AI problem. But having awareness of the biases will make our content more inclusive, and ultimately stronger and better.
To that end, we recently spoke with three AI experts who work with AI. Eleonore Fournier-Tombs works for the United Nations and is based in New York. Hessie Jones is a contributing writer to Forbes based in Toronto. Dr. Alice Martin works for the Clinton Health Access Initiative, Inc., and is based in Burkina Faso.
Studies show that when you have a more diverse set of people participating, contributing, and leading, you get better outcomes.
We focused on a specific confluence: AI and gender. Now, gender disparity in the tech sector isn’t exactly breaking news. But it is very real. Women hold just one-tenth of executive positions in Silicon Valley. Women founders receive a scant 2.3% of venture capital funding. Despite comprising nearly half of the US workforce, women are significantly underrepresented in the science, technology, engineering, and math (STEM) workforce — where they also earn less than their male counterparts.
There are copious, important reasons for gender parity in this field: social progress, economic growth, and innovation. For more on the challenges — and opportunities for women and AI — read our conversation below.
On acknowledging the role of women and AI
Eleonore Fournier-Tombs: In my own work, I’m focused on the recognition aspect — recognizing the work of women in AI.
Hessie Jones: Women continue to be largely underrepresented in leadership; our voices drowned out and our opinions dismissed. We need to start surfacing the people who have something important to say, and who have done the work. But many of them remain hidden because they don’t have star status.
Dr. Alice Martin: This past December 2023, the New York Times released a list of the top 10 personalities in the AI field. There were absolutely no women in the list, even while there are many accomplished women with lots of responsibilities in that field. So when I think about “women and AI,” the word that comes to mind is “underrepresented.” It’s a problem of diversity in general. And that has a huge impact on the kind of innovation being brought to the table.
On AIs reflecting societal bias
HJ: When LLMs scrape data that’s already biased, they absorb that bias. Take image generators: they’re full of specific biases and reflect preconceived notions and perceptions. It’s not just male-centric, but also Western-led since lots of the tech comes from here. But what they’re defining as XYZ is the definition that ends up prevailing.
I recently asked an image generator to tell me what an average person looks like in Brampton, a diverse city in Ontario with a large South Asia population. It showed me Indian men, no women. I put in the same prompt but a different city, Waterloo. It showed me a student who was male, and three older white males. No women. There’s a clear imbalance in the data set, and it’s reflecting the unconscious biases that humans have.
The good news is once you know that your data is biased, there are ways to correct it. But how can you know it’s biased?
AM: I once worked on a data set of patients with neurocognitive disorders. We were trying to predict the evolution of the disease, and determine the kind of care patients would need. We had a score that evaluates patient autonomy in everyday tasks: cooking, cleaning, bills. The scoring, designed in the 1970s by a group of men, had the tendency to declare men as less autonomous than women because a lot of men in their 80s (the kind of patients who have neurocognitive disorders) did not clean or cook. But we realized that it wasn’t because they couldn’t perform these tasks. They were just used to somebody else doing it.
This had a huge impact because care was being recommended based on that score, and the resulting algorithm would favor men. So more men were getting that type of care, even when they may not have truly needed it.
HJ: When I worked in banking, we had a suppression list for credit cards. In Canada, we have social insurance numbers (SIN), equivalent to the American Social Security number (SSN). If your SIN starts with 999, that indicates you’re a newcomer to the country. And our system would automatically flag all 999 SINs as a credit risk.
It didn’t matter if you had a really good income, you were automatically labeled a risk. That was a big aha moment for me: People were making decisions based on a system, but there was a bias embedded in that system. People were deemed not creditworthy, and their lives would be affected: You ended up with a higher interest rate, carrying a monthly balance, and going further into debt. Then the bank could say: “See, you’ve defaulted on your credit card. See, we’re going to keep rejecting people like you.”
We were able to correct this score. But that’s an example of the power of data, and what happens when the data is impacted by our human biases.
AM: The good news is once you know that your data is biased, there are ways to correct it. But how can you know it’s biased? There are no standards today that say, do this, this, and that to make sure the algorithm within your AI product is not biased.
From my perspective, there’s a lack of interest in addressing that very issue. We’ve put money into advancing and making these models effective and efficient, but less into safeguarding their use and their potential harm. Some say that will slow down innovation. But it’s important to have those limitations before we overstep them and then say, oops! We did it wrong.
EFT: That’s why diversity of thought is so beneficial. Studies show that when you have a more diverse set of people participating, contributing, and leading, you get better outcomes. When you can have good deliberations, you come up with good ideas.
Otherwise you’ll get groupthink, which can lead to missing major mistakes and limited thinking.
On possibilities and opportunities
HJ: Companies need to do bias mitigation at the source. That means creating representative data. Some companies are even creating synthetic data, which helps equalize the number of representations — not just for women and men, but all the different nuances so the models won’t over-index on specific areas.
At the end of the day, we want progress. But progress for everyone, not just for a select few.
Data privacy plays a huge part. We can pseudonymize information to get aggregated insights without risking the exposure of personal information. Because with models, we’re trying to get that aggregated view without biasing one specific population. We want model efficacy but need to balance the variables and reduce the ability for people to be identified. You have to balance out the personal information with the outcome you’re trying to get to. It’s like trying to reverse engineer something that’s biased. How do you un-bias it?
EFT: Freshness of ideas matters. When you have women, people of different ethnic backgrounds, different linguistic backgrounds — that opens up innovation.
On women and innovation
HJ: Education is critical. And it needs to go beyond equality and equity. It’s about upturning all the systems. But starting from ground zero isn’t realistic. You need to reverse engineer the bias. You need to un-bias, even if it’s little by little. At the end of the day, we want progress. But progress for everyone, not just for a select few. That means checks and balances. That means individual and corporate responsibility. We need to be able to uplift everyone.
EFT: There’s an old, false idea that women aren’t innovators in society. The reality is that for hundreds of years, women have significantly contributed to innovation. But they were usually uncredited. Many innovators were husband and wife duos. When they got published, when they got visibility, it was under the man’s name. Albert Einstein is a known genius. But nobody remembers his wife’s name, even though they worked together on many of his projects.
There’s also this phrase “godfathers of AI” — not godmothers. But while the CTO of OpenAI is probably one of the most important people in tech today, most people don’t know her name. (Ed. note: It’s Mira Murati, and she was Interim CEO of OpenAI for a brief minute.)
On what those who write about AI can do to help shine light on biases
EFT: When writing about AI, it’s important to not simply echo what’s already been said. That's particularly important when it comes to discussing risks and opportunities, and also when citing so-called “AI experts.” There’s been a lot of bias in amplifying the voices of only certain people in AI. Many pioneers, particularly women, have been less heard. Instead, take a balanced view and do additional research. And do not assume that what’s already been published on AI is unbiased.
HJ: Bias is a huge issue. As a writer, I look at how it came to be. I examine rules and processes that emerged in our banking and healthcare systems. I turn the lens onto how intersectionality, inequity, and deeply personal experiences have shaped stereotypes, which have then been baked into our systems. I interview experts who research alignment, technological determinism, the digital divide — all of which may remediate bias, or make it worse.
AI has its roots in history, in what was once acceptable practice. This model of the world continues to be shaped by the media. We need to ensure that we present a counternarrative that informs how the status quo came to be — and how we can change it.
Eleonore Fournier-Tombs is a senior data scientist and researcher. She is head of Anticipatory Action and Innovation at United Nations University - Centre for Policy Research (UNU-CPR), and author of Gender Reboot: Reprogramming Gender Rights in the Age of AI. She is based in New York, New York.
Hessie Jones is a digital and privacy strategist, a data rights advocate, and a tech journalist. As a contributing writer to Forbes, she profiles thinkers, startup innovators, and organizations up to disruptive and transformative things in data and AI. She is based in Toronto, Ontario, Canada.
Dr. Alice Martin is a health financing manager who focuses on pioneering innovations in the healthcare sector, especially within AI. She is a Health System Strengthening and Financing program manager at the Clinton Health Access Initiative, Inc., based in Burkina Faso.
About the author
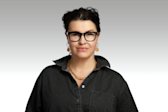
Justine Jablonska
Justine is a writer and editor who has lived and worked in Chicago, Warsaw, Washington, D.C., and now New York. She's spent her career creating award-winning content on a broad range of topics, including technology, diplomacy and government, business, and DE&I. In her previous stints on publishing teams at McKinsey, IBM, Boeing, and the Embassy of Poland in the U.S., she conceived and created content in a multitude of formats, including deeply reported stories, multimedia features, data-driven digital reports, videos, and newsletters. Her creative and freelance writing has appeared on Zocalo Public Square, Calvert Journal, and Cosmopolitan Review, among others. She holds a master's degree from Northwestern University's Medill School of Journalism.